U. researchers develop machine learning model to predict severity of coronavirus cases
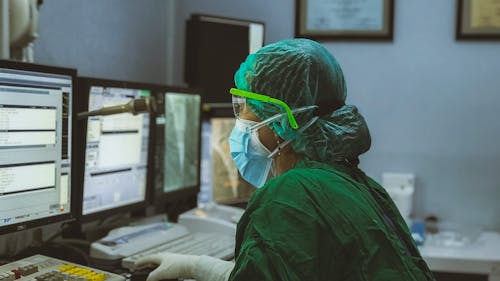
Recently, a team of Rutgers researchers produced a six-factor model that predicts the likelihood of death in a given case of coronavirus disease (COVID-19) using machine-learning software, according to Rutgers Health.
In the study, the researchers concluded that hospitals can use six factors to predict the prognosis of a patient's case. The six variables include platelet count, lactate, age, blood urea nitrogen, aspartate aminotransferase and C-reactive protein (PLABAC).
David Natanov, a Robert Wood Johnson Medical School (RWJMS) student and the study's lead author, said the project was inspired by the abnormality of COVID-19's effects compared to other respiratory viruses.
"Unlike other diseases like the flu and other respiratory bugs, COVID-19 was causing a lot of weird problems to occur, including kidney failure, liver damage, weird things that other respiratory bugs normally wouldn't cause," he said.
Regarding previous studies on COVID-19, Natanov said they often relied on technology that was not always readily available in hospital labs. Early on in the pandemic, hospitals were using the CURB-65 model for pneumonia, but there was a need for an efficient, accessible COVID-19-specific model, he said.
Natanov and other researchers collected data from more than 900 cases at Robert Wood Johnson University Hospital (RWJUH) at the height of the pandemic, he said.
After testing several models, including an initial 77-variable model, he said PLABAC and a similar six-factor model, platelet count, red blood cell distribution width, age, blood urea nitrogen, lactate and eosinophil count (PRABLE), rose as the most predictive set of variables.
For hospitals, Natanov said this model would allow incoming patients to be objectively analyzed upon admission, allowing physicians to give care tailored to a patient's specific case. He said an efficient system is particularly valuable in case of a new variant.
"If one patient who you think is not that sick actually has really, really high odds of mortality per the PLABAC model, maybe you give them an extra look," he said. "You spend that extra few minutes checking their chart, making sure you're not missing something."
Byron Avihai, a doctoral candidate at RWJMS—Princeton and a researcher on Natanov's team, said the project gave him and other medical students extensive experience in large-scale data collection and patient chart analysis, respectively.
Additionally, he said the team's data collection method, which took a year and a half to develop, could be applied to any disease. In the future, he said researchers could expand the study to observe long COVID and its determining factors.
Like Natanov, Avihai said the implications of the project are particularly valuable for hospitals where COVID-19 currently stands at a manageable rate. Yet, health systems have a history of becoming overwhelmed, especially when outbreaks of sicknesses like the flu, COVID-19 and RSV overlap, as seen late last year.
Even during this less severe point in the disease's timeline, Avihai said not protecting oneself from COVID-19 remains dangerous due to its unpredictability.
"We don't know what's going to happen in the future of COVID-19," Avihai said.
Both Natanov and Avihai said the virus constantly mutates, which makes it important for health care providers to track it closely. Avihai talked about the Omicron variant's unique tendency to target pregnant people more than previous iterations of the disease.
Natanov said he hopes the PLABAC model can be implemented in hospitals for individuals to see their family members' conditions.
"We're continuing to study the way that COVID-19 exists as a clinical entity, and from that, we can be able to more accurately assess who's going to be really sick and not," he said. "This model can be used for good in a lot of different places."